In the rapidly evolving world of artificial intelligence (AI), one element stands out as the cornerstone of success: quality data. As businesses rush to adopt AI technologies, many overlook the critical importance of the data that fuels these systems in the first place. This oversight can lead to disappointing results and wasted potential.
In this post, we'll explore why quality data is essential for AI success and how businesses can ensure they're building on a solid foundation.
The Role of Data in AI
AI systems, at their core, are really just sophisticated pattern recognition tools that learn from vast amounts of data to identify trends, make predictions, and automate decision-making processes.
However, the old adage "garbage in, garbage out" holds especially true when it comes to AI. The quality of your AI's output is directly proportional to the quality of data you feed into it.
Why Quality Data Matters
- Accuracy and Reliability: High-quality data leads to more accurate predictions and insights. Simple. Poor data can result in flawed decisions that could harm your business.
- Bias Mitigation: Quality data helps reduce algorithmic bias, ensuring fair and ethical AI applications.
- Efficiency: Clean, well-structured data allows AI systems to process information more efficiently, saving time and computational resources.
- Scalability: As your AI initiatives grow, the importance of quality data compounds. Starting with a solid data foundation makes scaling easier and more effective.
The Consequences of Poor Data Quality
- Inaccurate Insights: AI models trained on flawed data will produce unreliable results, potentially leading to costly business mistakes that could have been prevented with a successful bottom-up approach at the outset.
- Wasted Resources: Time and money spent on AI initiatives can be squandered if the underlying data is poor.
- Regulatory Risks: In industries with strict data regulations such as in Healthcare or Finance, poor data quality can lead to compliance issues and legal repercussions.
- Lost Opportunities: Inaccurate AI predictions can cause businesses to miss out on valuable opportunities or make ill-informed strategic decisions.
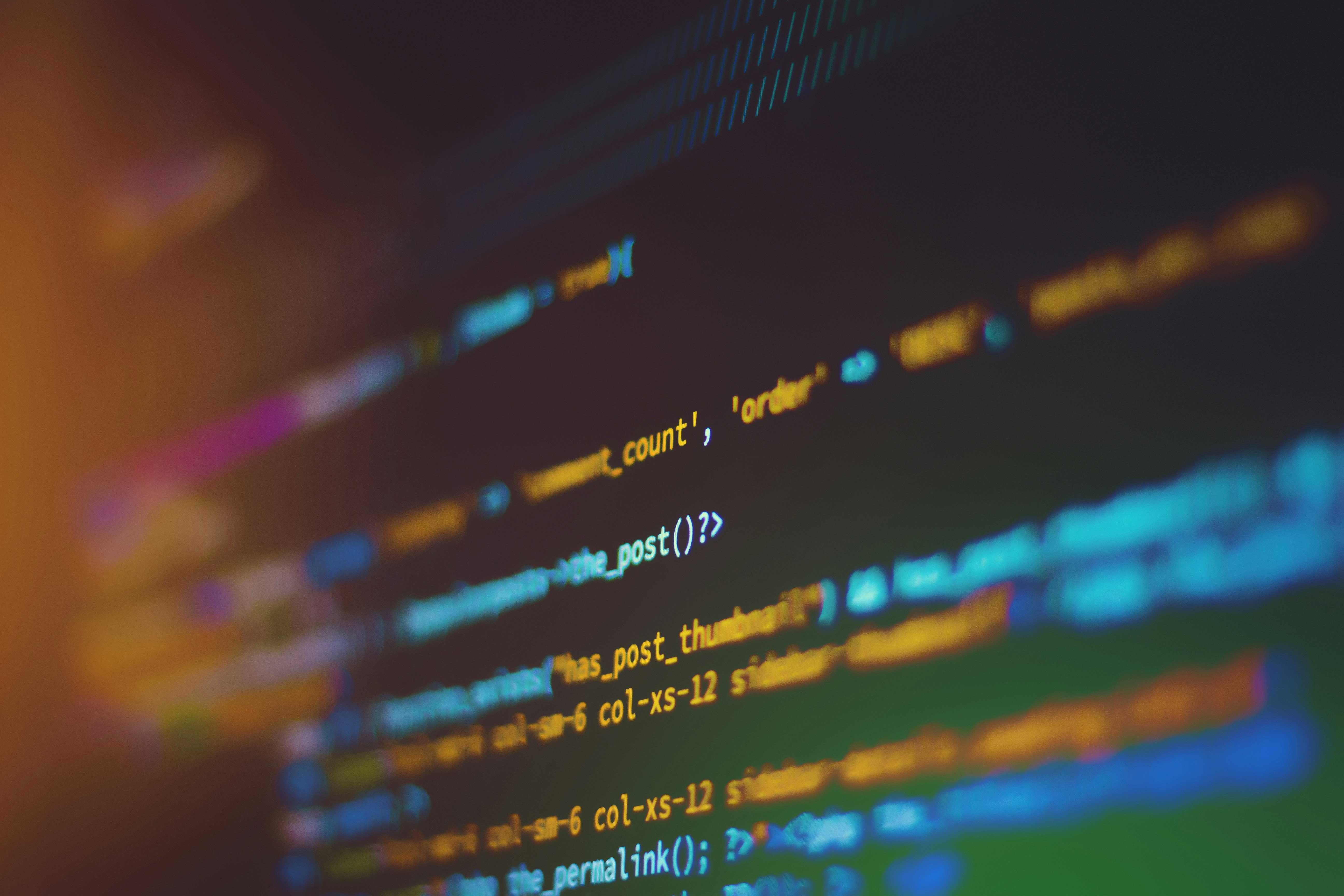
How to Ensure Data Quality for AI Success
- Implement Data Governance: Establish clear policies and procedures for data collection, storage, and management.
- Invest in Data Cleaning: Regularly clean and preprocess your data to remove errors, inconsistencies, and duplicates.
- Validate Data Sources: Ensure that your data comes from reliable and diverse sources to avoid bias and improve representativeness.
- Continuous Monitoring: Implement systems to continuously monitor data quality and flag issues as they arise.
- Train Your Team: Educate your staff on the importance of data quality and best practices for data handling.
- Use Data Quality Tools: Leverage specialized software tools like designed to assess and improve data quality.
Conclusion
In the race to adopt AI, the quality of your data can be the difference between success and failure. By prioritizing data quality, businesses can unlock the true potential of AI, gaining accurate insights, improving decision-making, and driving innovation at scale.
“Invest in quality data, and you'll be laying the groundwork for future AI-powered success.”